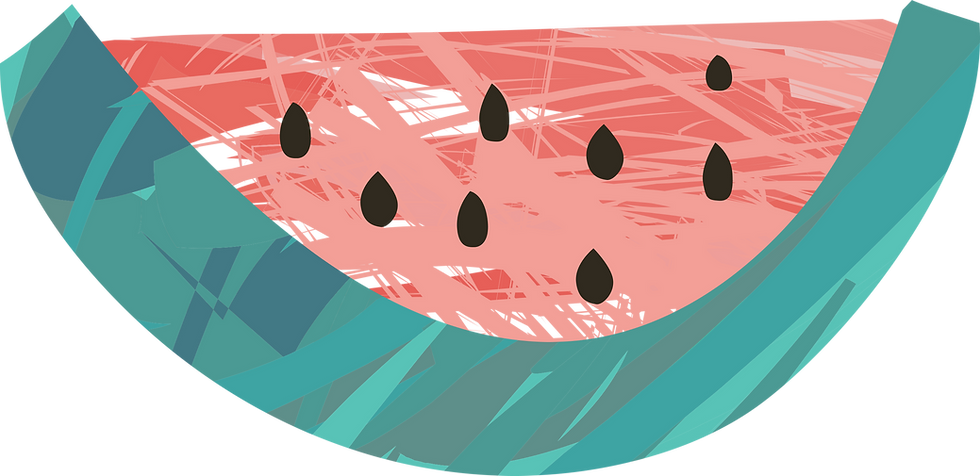
In the last blog, I touched on the role of the Product Manager in bringing stakeholders together to understand the process of building AI health tech.
Given the different types of end-users and the infrastructure that the AI product will eventually sit in, I proposed the need for user-centric design.
In this piece, I will talk through an example where my team and I have benefited from user-centric design, specifically where co-design has helped.
Problem Statement
The challenge was to transform a risk stratification AI model into a practical, user-centric software solution for chemotherapy decision support.
The clinical lead had a clear idea of the current chemotherapy pathway and how this product would be beneficial but had not yet fully explored the clinical impact or patient experience from this software.
Solution Exploration
Care Pathway Mapping: We analyzed the existing care pathway to work out where the product would have the most impact, taking care to include all end users (consultants, pharmacists, nurses, health care practitioners and patients) and technical integration needs.
User Interviews: Interviews with clinicians and focus groups with patients were conducted to gather in-depth insights into their needs and concerns.
Prototype Development: Based on the research findings, we developed prototypes of a clinical front-end for the decision support software. These were presented in stakeholder workshops for feedback and consensus.
Key Findings and Insights
Clinical Pathway Impact: The AI software had the potential to improve care by prioritizing high-risk patients and enabling self-care for low-risk patients.
Acceptability: Patients felt the use of a decision support tool was acceptable as long as the clinical team had the final decision. Clinicians also found the tool acceptable as part of their work but had caveats. They didn’t want another tick-box exercise.
Design Validation: User testing of the prototypes demystified the AI - end-users had a better understanding of how it could impact them and how they wanted to interact with it.
As part of this work, we recommended a new care pathway where the AI software would provide the most benefit to end users. Workshops with end users validated this and we were now in a position where we weren’t only offering a new product but also suggesting a change to the clinical pathway. End users agreed with this, but this would not be easy to adopt nationally as it would involve clinical directors, trusts and national bodies’ agreement.
Conclusion
So, after all this, what did we learn? Well, it turns out that just throwing AI at a problem isn't enough. You've gotta get people involved from the start. Talking to clinicians, nurses, and patients not only helped us understand what they needed. We also found that the way things were done in the hospital needed to change a bit to make the most of our AI tool.
And patients? They wanted more control and more info. They were less concerned with a clinical decision support tool that they’d not directly interact with. In this example, we tailored to the who the tool would impact. Although patients are also end-users of the chemotherapy service that this software would be a part of, their limited interaction meant we checked with them but didn’t need to involve them as part of the design of the solution. Being explicit about this and agreeing with end users builds the trust that can help your product get adopted by real users because they can see it's made with real user input.
User-centric design helped identify who the critical users were and what their user needs were as part of the clinical pathway mapping. We worked with users to better understand the implementation: how the tool could be best used in the real world, led to an improved co-designed solution. This would need more evidence and collaboration with organisations to explore the impact on the wider infrastructure. Without this, there’s a risk that your product becomes a tick-box exercise.
This might suggest a problem-first approach could help identify these opportunities earlier. In the next blog, I will explore another case study where we did take this approach: the AI models were developed after we delved into the user needs.